Chapter 9 Fractional factorial designs
9.1 Introduction
Factorial treatment designs are necessary for estimating factor interactions and offer additional advantages (Chapter 6). However, their implementation is challenging if we consider many factors or factors with many levels, because the number of treatments then might require prohibitive experiment sizes. Large factorial experiments also pose problems for blocking, since reasonable block sizes that ensure homogeneity of the experimental material within a block are often smaller than the number of treatment level combinations.
For example, a factorial treatment structure with five factors of two levels each already has \(2^5=32\) treatment combinations. An experiment with 32 experimental units then has no residual degrees of freedom, but two full replicates of this design already require 64 experimental units. If each factor has three levels, the number of treatment combinations increases drastically to \(3^5=243\).
On the other hand, we can often justify the assumption of effect sparsity: effect sizes of high-order interactions are often negligible, especially if interactions of lower orders already have small effect sizes. The key observation for reducing the experiment size is that a large portion of model parameters relate to higher-order interactions: in our example, there are 32 model parameters: one grand mean, five main effects, ten two-way interactions, ten three-way interactions, five four-way interactions, and one five-way interaction. The number of higher-order interactions and their parameters grows fast with increasing number of factors as shown in Table 9.1 for factorials with two factor levels and 3 to 7 factors.
If we ignore three-way and higher interactions in the example, we remove 16 parameters from the model equation and only require 16 observations for estimating the remaining model parameters; this is known as a half-fraction of the \(2^5\)-factorial. Of course, the ignored interactions do not simply vanish, but their effects are now confounded with those of lower-order interactions or main effects. The question then arises: which 16 out of the 32 possible treatment combinations should we consider such that no effect of interest is confounded with a another non-negligible effect?
Factorial | 0 | 1 | 2 | 3 | 4 | 5 | 6 | 7 |
---|---|---|---|---|---|---|---|---|
3 | 1 | 3 | 3 | 1 | ||||
4 | 1 | 4 | 6 | 4 | 1 | |||
5 | 1 | 5 | 10 | 10 | 5 | 1 | ||
6 | 1 | 6 | 15 | 20 | 15 | 6 | 1 | |
7 | 1 | 7 | 21 | 35 | 35 | 21 | 7 | 1 |
In this chapter, we discuss the general construction and analysis of fractional replications of \(2^k\)-factorial designs where all factors have two levels. This restriction is often sufficient for practical experiments with many factors, where interest focuses on identifying relevant factors and low-order interactions. We first consider generic factors which we call A, B and so forth, and denote their levels as low (or \(-1\)) and high (or \(+1\)). Similar techniques to those discussed here are available for factorials with more than two factors levels and for combination of factors with different number of levels, but the required mathematics is beyond our scope.
We further extend our ideas of fractional replication to deliberately confound some effects with blocks. This allows us to run a \(2^5\)-factorial in blocks of size 16, for example. By altering the confounding between pairs of blocks, we can still recover all effects, albeit with reduced precision.
9.2 Aliasing in the \(2^3\) factorial
9.2.1 Introduction
We begin our discussion with the simple example of a \(2^3\)-factorial treatment structure in a completely randomized design. We denote the treatment factors A, B, and C and their levels as \(A\), \(B\), and \(C\) with values \(-1\) and \(+1\). Recall that for any \(2^k\)-factorial, all main effects and all interaction factors (of any order) have one degree of freedom. We can thus also encode the two independent levels of any interaction as \(-1\) and \(+1\), and we define the level by multiplying the levels of the constituent factors: for \(A=-1\), \(B=+1\), \(C=-1\), the level of A:B is \(AB=A\cdot B=-1\) and the level of A:B:C is \(ABC=A\cdot B\cdot C=+1\).
It is also convenient to use an additional shorthand notation for a treatment combination, where we use a character string containing the lower-case letter of a treatment factor if it is present on its high level, and no letter if it is present on its low level. For example, we write \(abc\) if A, B, C are on level \(+1\), and all potential other factors are on the low level \(-1\), and \(ac\) if A and C are on the high level, and B on its low level. We denote a treatment combination with all factors on their low level by \((1)\). For a \(2^3\)-factorial, the eight different treatments are then \((1)\), \(a\), \(b\), \(c\), \(ab\), \(ac\), \(bc\), and \(abc\).
For example, testing compositions for growth media with factors Carbon with levels glucose
and fructose
, Nitrogen with levels low
and high
, and Vitamin with levels Mix 1
and Mix 2
leads to a \(2^3\)-factorial with the 8 possible treatment combinations shown in Table 9.2.
C-Source | Nitrogen | Vitamin | A | B | C | AB | AC | BC | ABC | Shorthand |
---|---|---|---|---|---|---|---|---|---|---|
glucose |
low |
Mix 1 |
\(-1\) | \(-1\) | \(-1\) | \(+1\) | \(+1\) | \(+1\) | \(-1\) | \((1)\) |
glucose |
low |
Mix 2 |
\(-1\) | \(-1\) | \(+1\) | \(+1\) | \(-1\) | \(-1\) | \(+1\) | \(c\) |
glucose |
high |
Mix 1 |
\(-1\) | \(+1\) | \(-1\) | \(-1\) | \(+1\) | \(-1\) | \(+1\) | \(b\) |
glucose |
high |
Mix 2 |
\(-1\) | \(+1\) | \(+1\) | \(-1\) | \(-1\) | \(+1\) | \(-1\) | \(bc\) |
fructose |
low |
Mix 1 |
\(+1\) | \(-1\) | \(-1\) | \(-1\) | \(-1\) | \(+1\) | \(+1\) | \(a\) |
fructose |
low |
Mix 2 |
\(+1\) | \(-1\) | \(+1\) | \(-1\) | \(+1\) | \(-1\) | \(-1\) | \(ac\) |
fructose |
high |
Mix 1 |
\(+1\) | \(+1\) | \(-1\) | \(+1\) | \(-1\) | \(-1\) | \(-1\) | \(ab\) |
fructose |
high |
Mix 2 |
\(+1\) | \(+1\) | \(+1\) | \(+1\) | \(+1\) | \(+1\) | \(+1\) | \(abc\) |
9.2.2 Effect estimates
In a \(2^k\)-factorial treatment structure, we estimate main effects and interactions as simple contrasts by subtracting the sum of responses of all observations with the corresponding factors on the low level from those with the factors on the high level. For our example, we estimate the main effect of C-Source (or generically A) by subtracting all observations with fructose
as our carbon source from those with glucose
, and averaging:
\[\begin{align*}
\text{A main effect} &= \frac{1}{4}\left(\,(a-(1)) + (ab-b) + (ac-c) + (abc-bc)\,\right) \\
&= \frac{1}{4}\left(\underbrace{(a+ab+ac+abc)}_{A=+1}-\underbrace{((1)+b+c+bc)}_{A=-1}\right)\;.
\end{align*}\]
A two-way interaction is a difference of differences and we find the interaction of B with C by first finding the difference between them for A on the low level and for A on the high level:
\[
\frac{1}{2}\underbrace{\left((abc-ab)\,-\,(ac-a)\right)}_{A=+1}
\quad\text{and}\quad
\frac{1}{2}\underbrace{\left((bc-b)\,-\,(c-(1))\right)}_{A=-1}\;.
\]
The interaction effect is then the averaged difference between the two
\[\begin{align*}
\text{B:C interaction} &= \frac{1}{4} \left(\;\left((abc-ab)-(ac-a)\right)+\left((bc-b)-(c-(1))\right)\;\right) \\
&= \frac{1}{4} \left(\; \underbrace{(abc+bc+a+(1))}_{BC=+1}\,-\,\underbrace{(ab+ac+b+c)}_{BC=-1}\; \right)\;.
\end{align*}\]
This value is equivalently found by taking the difference between observations with \(BC=+1\) (the interaction at its ‘high’ level) and \(BC=-1\) (the interaction at its ‘low’ level) and averaging. The other interaction effects are estimated by contrasting the corresponding observations for \(AB=\pm 1\) and \(AC=\pm 1\), and \(ABC=\pm 1\), respectively.
9.2.3 Design with four treatment combinations
We are interested in reducing the size of the experiment and for reasons that will become clear shortly, we choose a design based on measuring the response for four out of the eight treatment combinations. This will only allow estimation of four parameters in the linear model, and exactly which parameters can be estimated depends on the treatments chosen. The question then is: which four treatment combinations should we select?
We investigate three specific choices to get a better understanding of the consequences for effect estimation. The designs are illustrated in Figure 9.1, where treatment level combinations form a cube with eight vertices, from which four are selected in each case.
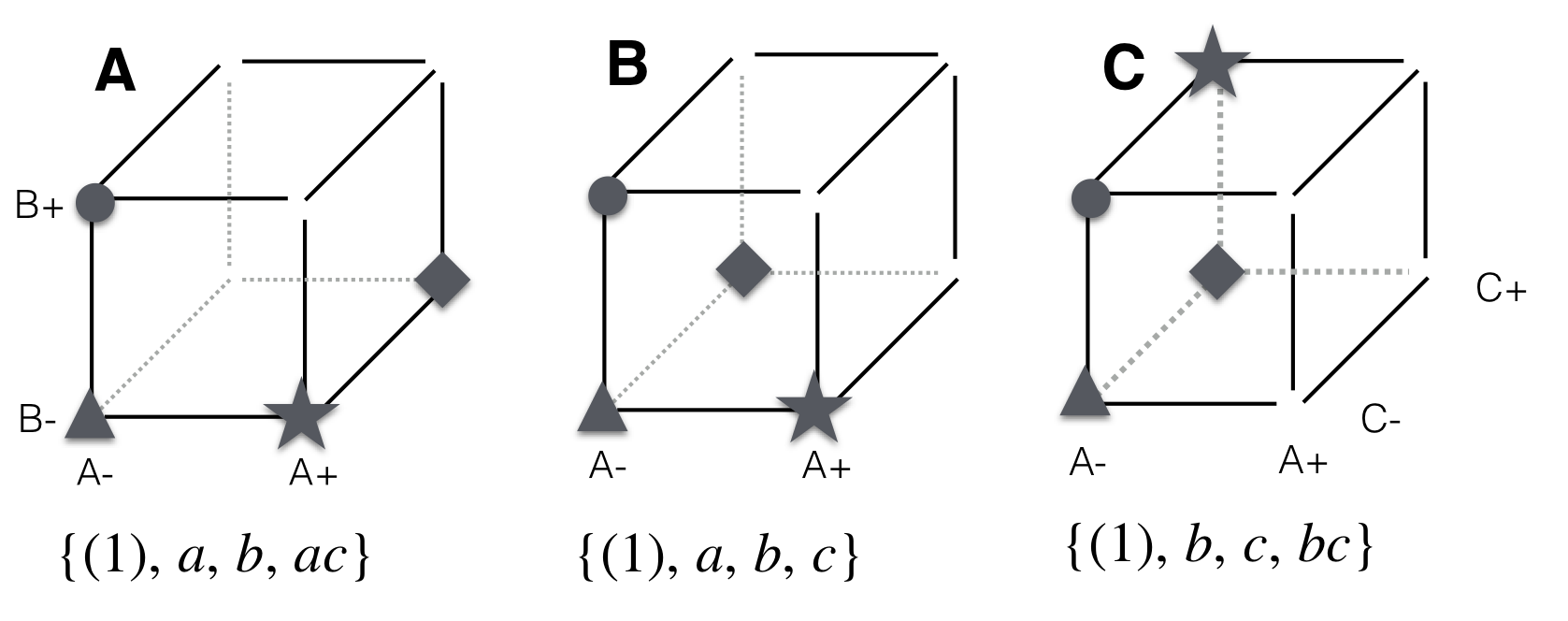
Figure 9.1: Some fractions of a \(2^3\)-factorial. A: Arbitrary choice of treatment combinations leads to problems in estimating any effects properly. B: One variable at a time (OVAT) design. C: Keeping one factor at a constant level confounds this factor with the grand mean and creates a \(2^2\)-factorial of the remaining factors.
First, we arbitrarily select the four treatment combinations \((1), a, b, ac\) (Fig. 9.1A). With this choice, none of the main effects or interaction effects can be estimated using all four data points. For example, an estimate of the A main effect involves \(a-(1)\), \(ab-b\), \(ac-c\), and \(abc-bc\), but only one of these—\(a-(1)\)—is available in this experiment. Compared to a factorial experiment in four runs, this choice of treatment combinations thus allows using only one-half of the available data for estimating this effect. If we would follow the above logic and contrast the observations with A at the high level with those with A at the low level, thereby using all data, the main effect is estimated as \((ac+a)-(b+(1))\) and obviously leads to a biased and incorrect estimate of the main effect, since the other factors are at ‘incompatible’ levels. Similar problems arise for B and C main effects, where only \(b-(1)\), respectively \(ac-a\) are available. None of the interactions can be estimated from these data and we are left with a very unsatisfactory muddle of conditional effect estimates that are valid only if other factors are kept at particular levels.
Next, we try to be more systematic and select the four treatment combinations \((1), a, b, c\) (Fig. 9.1C) where all factors occur on low and high levels. Again, main effect estimates are based on half of the data for each factor, but their calculation is now simpler: \(a-(1)\), \(b-(1)\), and \(c-(1)\), respectively. We note that each estimate involves the same level \((1)\). This design resembles a one variable at a time experiment, where effects can be estimated individually for each factor, by no estimates of interactions are available. All advantages of a factorial treatment design are then lost.
Finally, we select the four treatment combinations \((1), b, c, bc\) with A on the low level (Fig. 9.1B). This design is effectively a \(2^2\)-factorial with treatment factors B and C and allows estimation of their main effects and their interaction, but no information is available on any effects involving the third treatment factor A. For example, we estimate the B main effect using \((bc+b)\,-\,(c+(1))\), and the B:C interaction using \((bc-b)-(c-(1))\). If we look more closely into Table 9.2, we find a simple confounding structure: the level of B is always identical to that of A:B. In other words, the two effects are completely confounded in this design, and \((bc+b)\,-\,(c+(1))\) is in fact an estimate of the sum of the B main effect and the A:B interaction. Similarly, C is completely confounded with A:C, and B:C with A:B:C. Finally, the grand mean is confounded with the A main effect; this makes sense since any estimate of the overall average is based only on the low level of A.
9.2.4 The half-replicate or fractional factorial
Neither of the previous three choices provided a convincing reduction of the factorial design. We now discuss a fourth possibility, the half-replicate of the \(2^3\)-factorial, called a \(2^{3-1}\)-fractional factorial. The main idea is to deliberately alias a high-order interaction with the grand mean. For a \(2^3\)-factorial, we alias the three-way interaction A:B:C by selecting either those four treatment combinations that have \(ABC=-1\) or those that have \(ABC=+1\). We call the corresponding equation the generator of the fractional factorial; the two possible sets are shown in Figure 9.2. With either choice, we find three more effect aliases by consulting Table 9.2. For example, using \(ABC=+1\) as our generator yields the four treatment combinations \(a, b, c, abc\) and we find that A is completely confounded with B:C, B with A:C, and C with A:B.
In this design, any estimate thus corresponds to the sum of two effects. For example, \((a+abc)-(b+c)\) estimates the sum of A and B:C: first, the main effect of A is found as the difference of the runs \(a\) and \(abc\) with A on its high level, and the runs \(b\) and \(c\) with A on its low level: \((a+abc)-(b+c)\). Second, we contrast runs with B:C on the high level (\(a\) and \(abc\)) with those with B:C on its low level (\(b\) and \(c\)) for estimating the B:C interaction effect, which is again \((a+abc)-(b+c)\).
The fractional factorial based on a generator deliberately aliases each main effect with a two-way interaction, and the grand mean with the three-way interaction. This yields a very simple aliasing of effects and each estimate is based on the full data. Moreover, we note that by pooling the treatment combinations over levels of one of the three factors, we create three different \(2^2\)-factorials based on the two remaining factors. For example, ignoring the level of C leads to the full factorial in A and B shown in Figure 9.2. This is a consequence of the aliasing, as C is completely confounded with A:B.
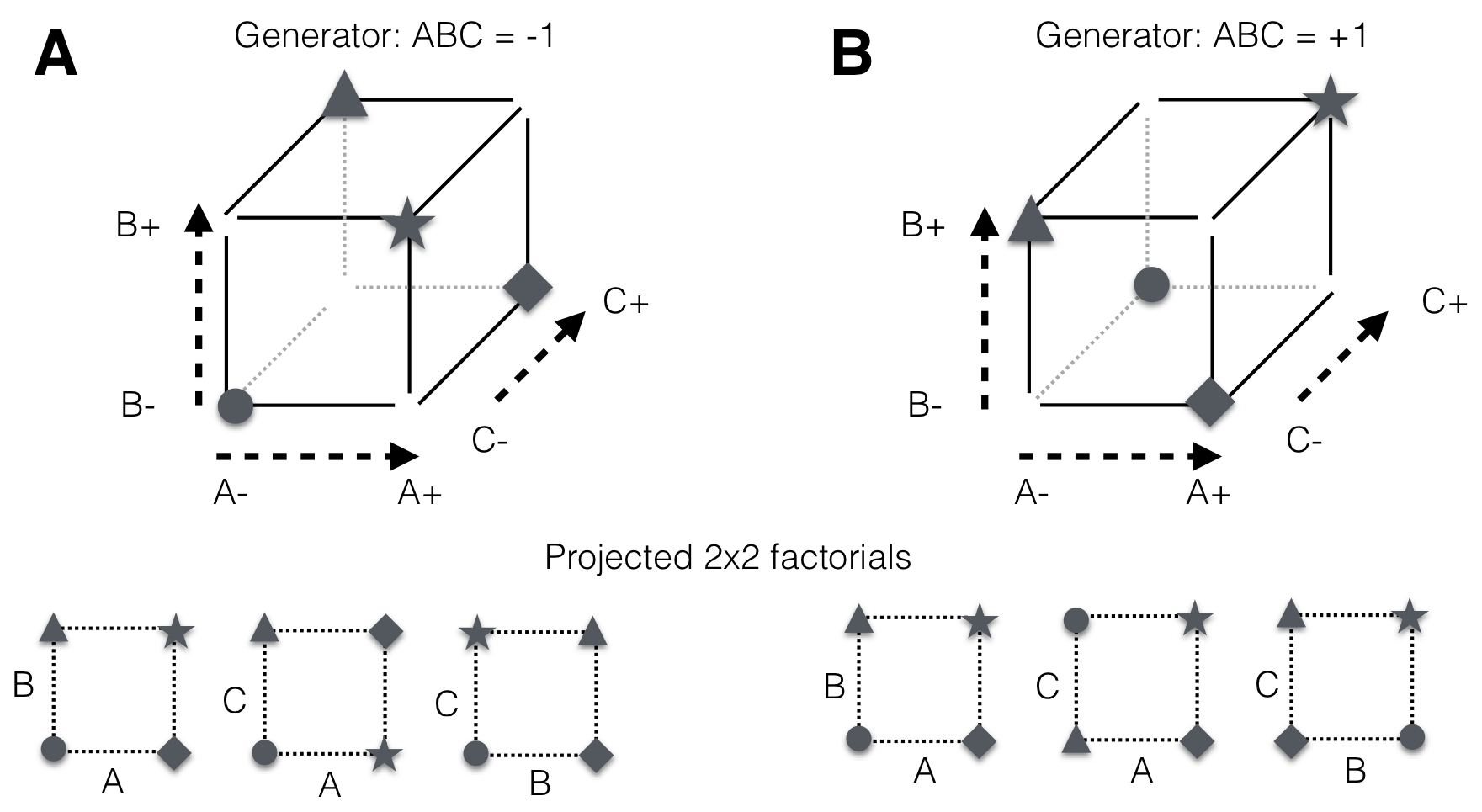
Figure 9.2: The two half-replicates of a \(2^3\)-factorial with three-way interaction and grand mean confounded. Any projection of the design to two factors yields a full \(2^2\)-factorial design and main effects are confounded with two-way interactions. A: design based on low level of three-way interaction; B: complementary design based on high level.
Our full linear model for a three-factor factorial is \[ y_{ijkl} = \mu + \alpha_i + \beta_j + \gamma_k + (\alpha\beta)_{ij} + (\alpha\gamma)_{ik} + (\beta\gamma)_{jk} + (\alpha\beta\gamma)_{ijk} + e_{ijkl} \] and it contains eight sets of parameters plus the residual variance. In a half-replicate of the \(2^3\)-factorial, we can only estimate the four derived parameters \[ \mu + (\alpha\beta\gamma)_{ijk}, \quad \alpha_i + (\beta\gamma)_{jk}, \quad \beta_j + (\alpha\gamma)_{ik}, \quad \gamma_k + (\alpha\beta)_{ij}\;. \] These provide the alias sets of confounded parameters, where only the sum of parameters in each set can be estimated: \[ \{1, ABC\}, \quad \{A, BC\}, \quad \{B, AC\}, \quad \{C, AB\}\;. \]
If the three interactions are negligible, then our four estimates correspond exactly to the grand mean and the three main effects. This corresponds to an additive model without interactions and allows a simple and clean interpretation of the parameter estimates. For example, with \((\beta\gamma)_{jk}=0\), the second derived parameter is now identical to \(\alpha_i\).
It might also be the case that the A and B main effects and their interaction are the true effects, while the factor C plays no role. The estimates of the four derived parameters are now estimates of the parameters \(\mu\), \(\alpha_i\), \(\beta_j\), and \((\alpha\beta)_{ij}\), while \(\gamma_k=(\alpha\gamma)_{ik}=(\beta\gamma)_{jk}=(\alpha\beta\gamma)_{ijk}=0\).
Many other combinations are possible, but the aliasing in the \(2^{3-1}\)-fractional factorial does not allow us to distinguish the different interpretations without additional experimentation.
9.3 Aliasing in the \(2^k\)-factorial
The half-replicate of a \(2^3\)-factorial does not provide an entirely convincing example for the usefulness of fractional factorial designs due to the complete confounding of main effects and two-way interactions, both of which are typically of great interest. With more factors in the treatment structure, however, we are able to alias interactions of higher order and confound low-order interactions of interest with high-order interactions that we might assume negligible.
9.3.1 Using generators
The generator or generating equation provides a convenient way for constructing fractional factorial designs. The generator is then a word written by concatenating the factor letters, such that \(AB\) denotes a two-way interaction, and our previous example \(ABC\) is a three-way interaction; the special ‘word’ \(1\) denotes the grand mean. A generator is then a formal equation that identifies two words and enforces the equality of the corresponding treatment combinations. In our \(2^{3-1}\) design, the generator \[ ABC=+1\;, \] selects all those rows in Table 9.2 for which the relation is true, i.e., for which \(ABC\) is on the high level.
A generator determines the effect confounding of the experiment: the generator itself is one confounding and \(ABC=+1\) describes the complete confounding of the the three-way interaction A:B:C with the grand mean.
From the generator, we can derive all other confoundings by simple algebraic manipulation. By formally ‘multiplying’ the generator with an arbitrary word, we find a new relation between effects. In this manipulation, the multiplication with the letter \(+1\) leaves the equation unaltered, multiplication with \(-1\) inverses signs, and a product of two identical letters yields \(+1\). For example, multiplying our generator \(ABC=+1\) with the word \(B\) yields \[ ABC\cdot B=(+1)\cdot B \iff AC=B\;. \] In other words, the B main effect is confounded with the A:C interaction. Similarly, we find \(AB=C\) and \(BC=A\) as two further confounding relations by multiplying the generator with \(C\) and \(A\), respectively.
Further trials with manipulating the generator show that no further relations can be obtained. For example, multiplying \(ABC=+1\) with the word \(AB\) yields \(C=AB\) again, and multiplying this relation with \(C\) yields \(C\cdot C=AB\cdot C\iff +1=ABC\), the original generator. This means that indeed, we have fully confounded four pairs of effects and no others. In general, a generator for a \(2^k\) factorial produces \(2^k/2=2^{k-1}\) such alias relations between factors, so we have a direct way to check if we found all. In our example, \(2^3/2=2^2=4\), so our alias relations \(ABC=+1\), \(AB=C\), \(AC=B\), and \(BC=A\) cover all existing confoundings.
This property also means that by choosing any of the implied relations as our generator, we get exactly the same set of treatment combinations. For example, instead of \(ABC=+1\), we might equally well choose \(A=BC\); this selects the same set of rows and implies the same set of confounding relations. Usually, we use a generator that aliases a high-order interaction with the grand mean, simply because it is the most obvious and convenient thing to do.
Useful fractions of factorial designs with manageable aliasing are associated with a generator, because then can effects be properly estimated and meaningful confounding arises. Each generator selects one-half of the possible treatment combinations and this is the reason why we set out to choose four rows for our examples, and not, say, six.
We briefly note that our first and second choice in Section 9.2.3 are not based on a generator, leaving us with a complex partial confounding of effects. In contrast, our third choice selected all treatments with A on the low level and does have a generator, namely \[ A=-1\;. \] Algebraic manipulation then shows that this design implies the additional three confounding relations \(AB=-C\), \(AC=-B\), and \(ABC=-BC\). In other words, any effect involving the factor A is confounded with another effect not involving that factor, which we easily verify from Table 9.2.
9.3.2 Half-fractions of higher \(2^k\) factorials
Generators and their algebraic manipulation provide an efficient way for finding the confoundings in higher-order factorials, where looking at the corresponding table of treatment combinations quickly becomes unfeasible. As we can see from the algebra, the most useful generator is always confounding the grand mean with the highest-order interaction.
For four factors, this generator is \(ABCD=+1\) and we expect that there are \(2^4/2=8\) relations in total. Multiplying with any letter reveals that main effects are then confounded with three-way interactions, such as \(ABCD=+1\iff BCD=A\) after multiplying with \(A\), and similarly \(B=ACD\), \(C=ABD\), and \(D=ABC\). Moreover, by multiplication with two-letter words we find that all two-way interactions are confounded with other two-way interactions, namely via the three relations \(AB=CD\), \(AC=BD\), and \(AD=BC\). This is already an improvement over fractions of the \(2^3\)-factorial, especially if we can make the argument that three-way interactions can be neglected and we thus have direct estimates of all main effects. If we find a significant and large two-way interaction—A:B, say—then we cannot distinguish if it is A:B, its alias C:D, or a combination of the two that produces the effect. Subject-matter considerations might be available to separate these possibilities. If not, there is at least a clear goal for a subsequent experiment to disentangle the two interaction effects.
Things improve further for five factors and the generator \(ABCDE=+1\) which reduces the number of treatment combinations from \(2^5=32\) to \(2^{5-1}=16\). Now, main effects are confounded with four-way interactions, and two-way interactions are confounded with three-way interactions. Invoking the principle of effect sparsity and neglecting the three- and four-way interactions yields estimable main effects and two-way interactions.
Starting from factorials with six factors, main effects and two-way interactions are confounded with interactions of order five and four, respectively, which in most cases can be assumed to be negligible.
A simple way for creating the design table of a fractional factorial using R
exploits these algebraic manipulations: first, we define our generator. We then create the full design table with \(k\) columns, one for each treatment factor, and one row for each of the \(2^k\) combinations of treatment levels, where each cell is either \(-1\) or \(+1\). Next, we create a new column for the generator and calculate its entries by multiplying the corresponding columns. Finally, we remove all rows for which the generator equation is not fulfilled and keep the remaining rows as our design table. For a 3-factor design with generator \(ABC=-1\), we create three columns \(A\), \(B\), \(C\) and eight rows. The new column \(ABC\) has entries \(A\cdot B\cdot C\), and we delete those rows for which \(A\cdot B\cdot C\not=-1\).
9.4 A real-life example: yeast medium composition
As a larger example of a fractional factorial treatment design, we discuss an experiment conducted during the sequential optimization of a yeast growth medium optimization. The overall aim was to find a medium composition that maximizes growth, and we discuss this aspect in more detail in Chapter 10. Here, we concentrate on determining the individual and combined effects of five medium ingredients—glucose Glc, two different nitrogen sources N1 (monosodium glutamate) and N2 (an amino acid mixture), and two vitamin sources Vit1 and Vit2—on the resulting number of yeast cells. Different combinations of concentrations of these ingredients are tested on a 48-well plate, and the growth curve is recorded for each well by measuring the optical density over time. We use the increase in optical density (\(\Delta\text{OD}\)) between onset of growth and flattening of the growth curve at the diauxic shift as a rough but sufficient approximation for increase in number of cells.
9.4.1 Experimental design
To determine how the five medium components influence the growth of the yeast culture, we used the composition of a standard medium as a reference point, and simultaneously altered the concentrations of the five components. For this, we selected two concentrations per component, one lower, the other higher than the standard, and considered these as two levels for each of five treatment factors. The treatment structure is then a \(2^5\)-factorial and would in principle allow estimation of the main effects and all two-, three-, four-, and five-factor interactions when all \(32\) possible combinations are used. However, a single replicate would require two-thirds of a plate and this is undesirable because we would like sufficient replication and also be able to compare several yeast strains in the same plate. Both requirements can be accommodated by using a half-replicate of the \(2^5\)-factorial with 16 treatment combinations, such that three independent experiments fit on a single plate.
A generator \(ABCDE=1\) confounds the main effects with four-way interactions, which we consider negligible for this experiment. Still, two-way interactions are confounded with three-way interactions, and in the first implementation we assume that three-way interactions are much smaller than two-way interactions. We can then interpret main effect estimates directly, and assume that derived parameters involving two-way interactions have only small contributions from the corresponding three-way interactions.
A single replicate of this \(2^{5-1}\)-fractional factorial generates 16 observations, sufficient for estimating the grand mean, five main effects, and the ten two-way interactions, but we are left with no degrees of freedom for estimating the residual variance. We say the design is saturated. This problem is circumvented by using two replicates of this design per plate. While this requires 32 wells, the same size as the full factorial, this strategy produces duplicate measurements of the same treatment combinations which we can manually inspect for detecting errors and aberrant observations. The 16 treatment combinations considered are shown in Table 9.3 together with the measured difference in OD for the first and second replicate, with higher differences indicating higher growth.
Glc | N1 | N2 | Vit1 | Vit2 | Growth_1 | Growth_2 |
---|---|---|---|---|---|---|
20 | 1 | 0 | 1.5 | 4 | 1.7 | 35.68 |
60 | 1 | 0 | 1.5 | 0 | 0.1 | 67.88 |
20 | 3 | 0 | 1.5 | 0 | 1.5 | 27.08 |
60 | 3 | 0 | 1.5 | 4 | 0.0 | 80.12 |
20 | 1 | 2 | 1.5 | 0 | 120.2 | 143.39 |
60 | 1 | 2 | 1.5 | 4 | 140.3 | 116.30 |
20 | 3 | 2 | 1.5 | 4 | 181.0 | 216.65 |
60 | 3 | 2 | 1.5 | 0 | 40.0 | 47.48 |
20 | 1 | 0 | 4.5 | 0 | 5.8 | 41.35 |
60 | 1 | 0 | 4.5 | 4 | 1.4 | 5.70 |
20 | 3 | 0 | 4.5 | 4 | 1.5 | 84.87 |
60 | 3 | 0 | 4.5 | 0 | 0.6 | 8.93 |
20 | 1 | 2 | 4.5 | 4 | 106.4 | 117.48 |
60 | 1 | 2 | 4.5 | 0 | 90.9 | 104.46 |
20 | 3 | 2 | 4.5 | 0 | 129.1 | 157.82 |
60 | 3 | 2 | 4.5 | 4 | 131.5 | 143.33 |
Clearly, the medium composition has a huge impact on the resulting growth, ranging from a minimum of 0 to a maximum of 181. The original medium has an average ‘growth’ of \(\Delta\text{OD}\approx 80\), and this experiment already reveals a condition with approximately 2.3 fold increase. We also see that measurement with N2 at the low level are abnormally low in the first replicate. We remove these eight values from our analysis.13
9.4.2 Analysis
Our fractional factorial design has five treatment factors and several interaction factors, and we use an analysis of variance initially to determine which of the medium components has an appreciable effect on growth, and how the components interact. The full model is growth~Glc*N1*N2*Vit1*Vit2
, but only half of its parameters can be estimated. Since we deliberately confounded effects in our fractional factorial treatment structure, we know which derived parameters are estimated, and can select one member of each alias set for our model. The model specification growth~(Glc+N1+N2+Vit1+Vit2)^2
asks for an ANOVA based on all main effects and all two-way interactions (it expands to growth~Glc+N1+N2+...+Glc:N1+...+Vit1:Vit2
). After pooling the data from both replicates and excluding the aberrant N2 observation of the first replicate, the resulting ANOVA table is
Df | Sum Sq | Mean Sq | F value | Pr(>F) | |
---|---|---|---|---|---|
Glc | 1 | 6148 | 6148 | 26.49 | 0.0008772 |
N1 | 1 | 1038 | 1038 | 4.475 | 0.0673 |
N2 | 1 | 34298 | 34298 | 147.8 | 1.94e-06 |
Vit1 | 1 | 369.9 | 369.9 | 1.594 | 0.2423 |
Vit2 | 1 | 6040 | 6040 | 26.03 | 0.0009276 |
Glc:N1 | 1 | 3907 | 3907 | 16.84 | 0.003422 |
Glc:N2 | 1 | 1939 | 1939 | 8.357 | 0.02017 |
Glc:Vit1 | 1 | 264.8 | 264.8 | 1.141 | 0.3166 |
Glc:Vit2 | 1 | 753.3 | 753.3 | 3.247 | 0.1092 |
N1:N2 | 1 | 0.9298 | 0.9298 | 0.004007 | 0.9511 |
N1:Vit1 | 1 | 1450 | 1450 | 6.248 | 0.03697 |
N1:Vit2 | 1 | 9358 | 9358 | 40.33 | 0.0002204 |
N2:Vit1 | 1 | 277.9 | 277.9 | 1.198 | 0.3057 |
N2:Vit2 | 1 | 811.4 | 811.4 | 3.497 | 0.0984 |
Vit1:Vit2 | 1 | 1280 | 1280 | 5.515 | 0.0468 |
Residuals | 8 | 1856 | 232 |
We find several substantial main effects in this analysis, with N2 the main contributor followed by Glc and Vit2. Even though N1 has no significant main effect, it appears in several significant interactions; this also holds to a lesser degree for Vit1. Several pronounced interactions demonstrate that optimizing individual components will not be a fruitful strategy, and we need to simultaneously change multiple factors to maximize the growth. This information can only be acquired by using a factorial design.
We do not discuss the necessary subsequent analyses of contrasts and effect sizes for the sake of brevity; they work exactly as for smaller factorial designs.
9.4.3 Alternative analysis of single replicate
Since the design is saturated, a single replicate does not provide information about uncertainty. If only the single replicate can be analyzed, we have to reduce the model to free up degrees of freedom from parameter estimation to estimate the residual variance. If subject-matter knowledge is available to decide which factors can be safely removed without missing important effects, then a single replicate can be a successfully analysed. For example, knowing that the two nitrogen sources and the two vitamin components do not interact, we might specify the model Growth~(Glc+N1+N2+Vit1+Vit2)^2 - N1:N2 - Vit1:Vit2
that removes the two corresponding interactions while keeping the three remaining ones. This strategy is somewhat unsatisfactory, since we now still only have two residual degrees of freedom and correspondingly low precision and power, and we cannot test if removal of the factors was really justified. Without good subject-matter knowledge, this strategy can give very misleading results if significant and large effects are removed from the analysis.
9.5 Multiple aliasing
The definition of a single generator creates a half-replicate of the factorial design. For higher-order factorials starting with the \(2^5\)-factorials, useful designs are also available for higher fractions, such as quarter-replicates that would require only 8 of the 32 treatment combinations in a \(2^5\)-factorial. These designs are constructed by using more than one generator, which also leads to more complicated confounding.
For example, a quarter-fractional requires two generators: one generator to specify one-half of the treatment combinations, and a second generator to specify one-half of those. Both generators introduce their own aliases which we determine using the generator algebra. In addition, multiplying the two generators introduces further aliases through the generalized interaction.
9.5.1 A generic \(2^{5-2}\) fractional factorial
As a first example, we construct a quarter-replicate of a \(2^5\)-factorial. Which two generators should we use? Our first idea is probably to use the five-way interaction for defining the first set of aliases, and one of the four-way interactions for defining the second set. We might choose the two generators \(G_1\) and \(G_2\) as \[ G_1: ABCDE=1 \quad\text{and}\quad G_2: BCDE=1\;, \] for example. The resulting eight treatment combinations are shown in Table 9.4(left). We see that in addition to the two generators, we also have a further highly undesirable confounding of the main effect of A with the grand mean: the column \(A\) only contains the high level. This is a consequence of the interplay of the two generators, and we find this additional confounding directly by comparing the left- and right-hand side of their generalized interaction: \[ G_1G_2 = ABCDE\cdot BCDE=ABBCCDDEE = A =1\;. \]
|
|
Some further trial-and-error reveals that no useful second generator is available if we confound the five-way interaction with the grand mean in our first generator. A reasonably good pair of generators uses two three-way interactions, such as \[ G_1: ABD=1 \quad\text{and}\quad G_2: ACE=1\;, \] with generalized interaction \[ G_1G_2 = AABCDE = BCDE = 1\;. \] The resulting treatment combinations are shown in Table 9.4(right). We note that main effects and two-way interactions are now confounded.
Finding good pairs of generators is not entirely straightforward, and software or tabulated designs are often used.14
9.5.2 A real-life \(2^{7-2}\) fractional factorial
The transformation of yeast cells is an important experimental technique, but many protocols have very low yield. In an attempt to define a more reliable and efficient protocol, seven treatment factors were considered in combination: Ion, PEG, DMSO, Glycerol, Buffer, EDTA, and amount of carrier DNA. With each component in two concentrations, the full treatment structure is a \(2^7\)-factorial with 128 treatment combinations. This experiment size is prohibitive since each treatment requires laborious subsequent steps, but 32 treatment combinations were considered reasonable for implementing this experiment. This requires a quarter-replicate of the full design.
Ideally, we want to find two generators that alias main effects and two-way interactions with interactions of order three and higher, but no such pair of generators exists in this case. We are confronted with the problem of confounding some two-way interactions with each other, while other two-way interactions are confounded with three-way interactions.
Preliminary experiments suggested that the largest interactions involve Ion, PEG, and potentially Glycerol, while the two-way interactions involving other components are all comparatively small. A reasonable design then uses the two generic generators \[ G_1: ABCDF=+1 \text{ and } G_2: ABDEG=+1 \] with generalized interaction \(CF=EG\). The two-factor interactions involving the factors C, E, F, and G are then confounded with each other, but two-way interactions involving the remaining factors A, B, and D are confounded with interactions of order three or higher. Hence, selecting A, B, D as the factors Ion, PEG, and Glycerol allows us to create a design with 32 treatment combinations that reflects our subject-matter knowledge and allows estimation of all relevant two-way interactions while confounding those two-way interactions that we consider negligible. For example, we cannot disentangle an interaction of DMSO and EDTA from an interaction of Buffer and carrier DNA, but this does not jeopardize the interpretation of this experiment.
9.6 Characterizing fractional factorials
Two measures to describe the severity of confounding in a fractional factorial design are the resolution and the abberration.
9.6.1 Resolution
A fractional factorial design has resolution \(K\) if the grand mean is confounded with at least one factor of order \(K\), and no factor of lower order. The order is typically given as a roman numeral. For example, a \(2^{3-1}\) design with generator \(ABC=1\) has order III, and we denote such a design as \(2^{3-1}_{\text{III}}\).
Designs with more factors allow fractions of higher resolution. Our \(2^5\)-factorial example in the previous section admits a \(2^{5-1}_{\text{V}}\) design with 16 combinations, and a \(2^{5-2}_{\text{III}}\) design with 8 combinations. With the first design, we can estimate main effects and two-way interactions free of other main effects and two-way interactions, while the second design aliases main effects with two-way interactions. Our 7-factor example has resolution IV.
For a factor of any order \(N\), the resolution also gives the lowest order of a factor confounded with it: a resolution-III design confounds main effects with two-way interactions (\(\text{III}=1+2\)), and the grand mean with a three-way interaction (\(\text{III}=0+3\)). A resolution-V design confounds main effects with four-way interactions (\(\text{V}=1+4\)), two-way interactions with three-way interactions (\(\text{V}=2+3\)), and the five-way interaction with the grand mean (\(\text{V}=5+0\)).
In general, resolutions \(\text{III}\), \(\text{IV}\), and \(\text{V}\) are the most ubiquitous, and a resolution of \(\text{V}\) is often the most useful if it is achievable, since then main effects and two-way interactions are aliased only with interactions of order three and higher. Main effects and two-way interactions are confounded for resolution III, and these designs are useful for screening larger numbers of factors, but usually not for experiments where relevant information is expected in the two-way interactions. If a design has many treatment factors, we can also construct fractions with resolution higher than V, but it is usually more practical to use an additional generator to construct a design with resolution V and fewer treatment combinations.
Resolution IV confounds two-way interaction effects with each other. While this is rarely desirable, we might find multiple generators that leave some two-way interactions unconfounded with other two-way interactions, as in our 7-factor example. Such designs offer dramatic decreases in the experiment size for large number of factors. For example, full factorials for nine, ten, and eleven factors have 512, 1024, and 2048 treatment combinations, respectively. For most experiments, this is clearly not practically implementable. However, fractional factorial of resolution IV only require 32 runs in each case, which is a very practical proposition in most situations.
Similarly, a \(2^{7-2}\) design has resolution IV, since some of the two-way interactions are confounded. The maximal resolutions for the \(2^7\) series are \(2^{7-1}_{VII}\), \(2^{7-2}_{IV}\), \(2^{7-3}_{IV}\), \(2^{7-4}_{III}\). Thus, the resolution drops with increasing fraction, and not all resolutions might be achievable for a given number of factors (there is no resolution-VI design for seven factors, for example).
9.6.2 Aberration
For the \(2^7\)-factorial, both a reduction by \(1/4\) and by \(1/8\) leads to a resolution-IV design, but these designs are clearly not comparable in other aspects. For example, all two-way interactions are confounded in the \(2^{7-3}\) design, while we saw that only some are confounded in the \(2^{7-2}\) design.
The abberration provides an additional criterion to compare designs with identical resolution and is found as follows: we write down the generators and derive their generalized interactions. We then sort the resulting set of alias relations by word length and count how many relations there are of each length. The fewer words of short length occur, the better the set of generators. This criterion thus encodes that we prefer aliasing higher-order interactions to aliasing lower-order interactions.
For the two \(2^7\) fractions of resolution IV, we find two relations of length four for the \(2^{7-2}\)-design, while there are seven such relations for the \(2^{7-3}\)-design. The confounding of the former is therefore less severe than the confounding of the latter.
The abberration can also be used to compare different sets of generators for the same fractional factorial. For example, the following two sets of generators both yield a \(2^{7-2}_{\text{IV}}\) design: \[ ABCDE=1,\,ABCEG=1 \quad\text{and}\quad ABCF=1\,,ADEG=1\;. \] The first set of generators has generalized interaction \(ABCDE\cdot ABCEG=DEFG=1\), so this design has a set of generating alias relations with one word of length four, and two words of length five. In contrast, the second set of generators has generalized interaction \(ABCF\cdot ADEG=BCDEFG=1\), and contains two words of length four and one word of length six. We would therefore prefer to use the first set of generators, because is yields a less confounded set of aliases.
9.7 Factor screening
A common problem, especially at the beginning of designing an assay or investigating any system, is to determine which of the vast number of possible factors actually have a relevant influence on the response. For example, let us say we want to design a toxicity assay with a luminescent readout on a 48-well plate, where luminescence is supposed to be directly related to the number of living cells in each well, and is thus a proxy for toxicity of a substance pipetted into a well. Apart from the substance’s concentration and toxicity, there are many other factors that one might imagine can influence the readout. Examples include the technician, amount of shaking before reading, the reader type, batch effects of chemicals, temperature, setting time, labware, type of pipette (small/large volume), and many others.
Before designing any experiment for more detailed analyses of relevant factors, we may want to conduct a factor screening to determine which factors are active and appreciably affect the response. Subsequent experimentation then only includes the active factors and, having reduced the number of treatment factors, can then be designed with the methods previously discussed.
Factor screening designs make extensive use of the assumption that the proportion of active factors among those considered is small. We usually also assume that we are only interested in the main effects and can ignore the interaction effects for the screening. This assumption is justified because we will not make any inference on how exactly the factors influence the response, but are for the moment only interested in discarding factors of no further interest.
9.7.1 Fractional factorials
One class of screening designs uses fractional factorial design of resolution \(\text{III}\). Noteworthy examples are the \(2^{15-11}_{\text{III}}\) design, which allows screening 15 factors in 16 runs, or the \(2^{31-26}_{\text{III}}\) design, which allows screening 31 factors in 32 runs!
A problem of this class of designs is that the ‘gap’ between useful screening design increases with increasing number of factors, because we can only consider fractions that are powers of two: reducing a \(2^7\) design with 128 runs yields designs of 64 runs (\(2^{7-1}\)) and 32 runs (\(2^{7-2}\)), but we cannot find designs with less than 64 and more than 32 runs, for example. On the other hand, fractional factorials are familiar designs that are relatively easy to interpret and if a reasonable design is available, there is no reason not to consider it.
Factor screening experiments will typically use a single replicate of the fractional factorial, and effects cannot be tested formally. If only a minority of factors is active, we can use a method by Lenth to still identify the active factors by more informal comparisons (Lenth 1989). The main idea is to calculate a robust estimate of the standard error and use it to discard factors whose effects are not sufficiently larger than this estimate.
Specifically, we denote the estimated average difference between low and high level of the \(j\)th factor by \(c_j\) and estimate the standard error as 1.5 times the median of absolute effect estimates: \[ s_0 = 1.5 \cdot \text{median}_{j} |c_j|\;. \] If no effect were active, then \(s_0\) would already provide an estimate of the standard error. If some effects are active, they inflate the estimate by an unknown amount. We therefore restrict our estimation to those effects that are ‘small enough’ and do not exceed 2.5 times the current standard error estimate. The pseudo standard error is then \[ \text{PSE} = 1.5 \cdot \text{median}_{|c_j|<2.5\cdot s_0} |c_j|\;. \] The margin of error (ME) (i.e., the upper limit of a confidence interval) is then \[ \text{ME} = t_{0.975, d} \cdot \text{PSE}\;, \] and Lenth proposes to use \(d=m/3\) as the degrees of freedom, where \(m\) is the number of effects in the model. This limit is corrected for multiple comparisons by adjusting the confidence limit from \(\alpha=0.975\) to \(\gamma=(1+0.95^{1/m})/2\). The resulting simultaneous margin of error (SME) is then \[ \text{SME} = t_{\gamma, d} \cdot \text{PSE}\;. \] Factors with effects exceeding SME in either direction are considered active, those between the ME limits are inactive, and those between ME and SME have unclear status. We therefore choose those factors that exceed SME as our safe choice, and might include those exceeding ME as well for subsequent experimentation.
In his paper, Lenth discusses a \(2^4\) full factorial experiment, where the effect of acid strength (S), time (t), amount of acid (A), and temperature (T) on the yield of isatin is studied (Davies 1954). The experiment design and the resulting yield are shown in Table 9.5.
|
|
The results are shown in Figure 9.3. No factor seems to be active, with temperature, acid strength, and the interaction of temperature and time coming closest.
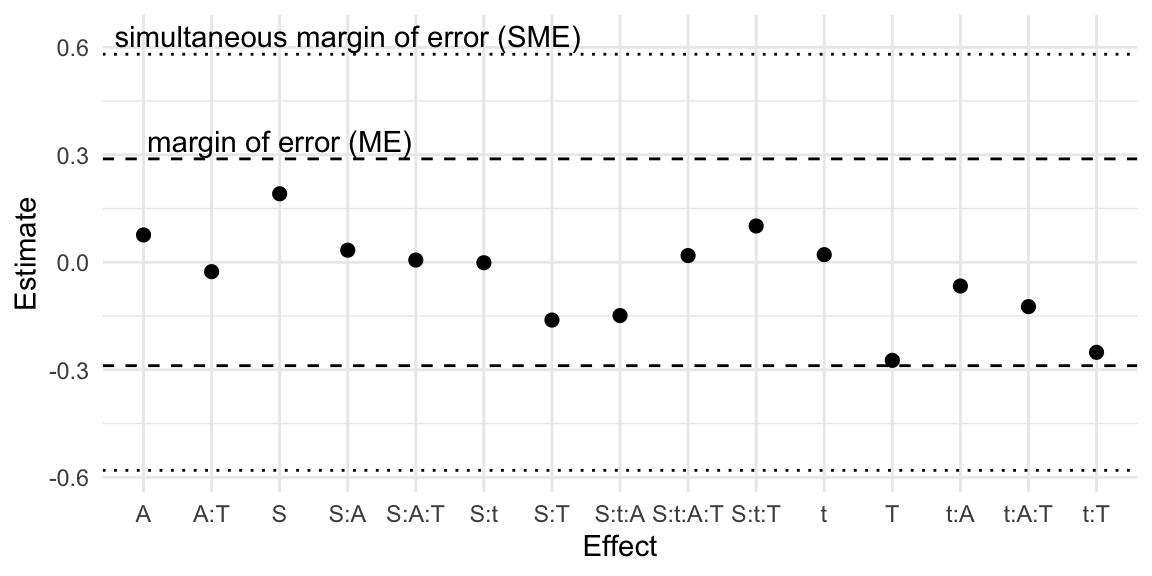
Figure 9.3: Analysis of active effects in unreplicated \(2^4\)-factorial with Lenth’s method.
9.7.2 Plackett-Burman designs
A different idea for constructing screening designs was proposed by Plackett and Burman in a seminal paper (Plackett and Burman 1946). These designs require that the number of runs is a multiple of four. The most commonly used are the designs in 12, 20, 24, and 28 runs, which can screen 11, 19, 23, and 27 factors, respectively. Plackett-Burman designs do not have a simple confounding structure that could be determined with generators. Rather, they are based on the idea of partially confounding some fraction of each effect with other effects. These designs are used for screening main effects only, as main effects are already confounded with two-way interactions in rather complicated ways that cannot be easily disentangled by follow-up experiments. Plackett-Burman designs considerably increase the available options for the experiment size, and offer several designs in the range of \(16, \dots, 32\) runs for which no fractional factorial design is available.
Tables of Plackett-Burman designs are found on the NIST website15 and in many older texts on experimental design. In R
, they can be constructed using the function pb()
from package FrF2
, which requires the number of runs \(n\) (a multiple of four) as its only input and returns a design for \(n-1\) factors.
9.8 Blocking factorial experiments
With many treatment, blocking a design becomes challenging because the efficiency of blocking deteriorates with increasing block size, or there are other limits on the maximal number of units per block. The incomplete block designs in Section 7.3 are a remedy for this problem for unstructured treatment levels. The ideas of fractional factorial designs is useful for blocking factorial treatment structures and explicitly exploit their properties by deliberately confounding (higher-order) interactions with block effects. This reduces the required block size to the size of the corresponding fractional factorial.
We can further extend this idea by using different confoundings for different sets of blocks, such that each set accommodates a different fraction of the same factorial treatment structure. We are then able to recover most of the effects of the full factorial, albeit with different precision.
We consider the \(2^3\)-factorial treatment structure as our main example, as it already allows discussion of all relevant ideas. We consider the case that our blocking factor only allows accommodating four out of the eight possible treatment combinations. This is a realistic scenario if studying combinations of three drug treatments on mice and blocking by litter, with typical litter sizes being below eight. Two questions arise: (i) which treatment combinations should we assign to the same block? and (ii) with replication of blocks, should we use the same assignment of treatment combinations to blocks? If not, how should we determine treatment combinations for sets of blocks?
9.8.1 Half-fraction
A first idea is to use a half-replicate of the \(2^3\)-factorial with four treatment combinations, and confound the generator with the block effect. If we use the generator \(ABC=+1\) and each block effect is confounded with the grand mean, so \(Block=+1\), then we get the formal generator \(ABC=Block\) and assign only those four treatment combinations with \(ABC=+1\) to each block. With four blocks, this yields the following assignment:
Block | Generator | 1 | 2 | 3 | 4 |
---|---|---|---|---|---|
I | ABC=+1 | a | b | c | abc |
II | ABC=+1 | a | b | c | abc |
III | ABC=+1 | a | b | c | abc |
IV | ABC=+1 | a | b | c | abc |
Within each block, we have the same one-half fraction of the \(2^3\)-factorial with runs \(\{a,b,c,abc\}\) and this design resembles a four-fold replication of the same fractional factorial, where systematic differences between replicates are accounted for by the block effects. The fractional factorial has resolution-\(\text{III}\), and main effects are confounded with two-way interactions.
From the 16 observations, we required four degrees of freedom for estimating the treatment parameters, and three degrees of freedom for the block effect, leaving us with nine residual degrees of freedom. The latter can be increased by using more blocks, where we gain four observations with each block, and loose one degree of freedom per block for the block effect. Since the effect aliases are the same in each block, increasing the number of blocks does not change the confounding an no matter how many block we use, we are unable to disentangle the main effect of A, say, and the B:C interaction in this design.
9.8.2 Half-fraction with alternating replication
We can improve the design substantially by noting that it is not required to use the same half-replicate in each block. Instead, we might use the generator \(ABC=+1\) with combinations \(\{(1),ab,ac,bc\}\) for two of the four blocks, and the corresponding generator \(ABC=-1\) (the fold-over) with combinations \(\{a,b,c,abc\}\) for the other two blocks. The design is then
Block | Generator | 1 | 2 | 3 | 4 |
---|---|---|---|---|---|
I | ABC=+1 | a | b | c | abc |
II | ABC=+1 | a | b | c | abc |
III | ABC=-1 |
|
ab | ac | bc |
IV | ABC=-1 |
|
ab | ac | bc |
With two replicates for each of the two levels of the three-way interaction, its parameters are estimable using the block totals. Somewhat loosely speaking, this resembles a split-unit design with A:B:C having blocks as experimental units, and all other effects randomized on units within blocks. All other effects can be estimated more precisely, since we now effectively have two replicates of the full factorial design after we account for the block effects. While the half-fraction of a \(2^3\)-factorial is not an interesting option in itself due to the severe confounding, it gives a very appealing design for reducing block sizes.
For example, we have confounding of A with B:C for observations based on the \(ABC=+1\) half-replicates (with \(A=BC\)), but we can resolve this confounding using observations from the other half-replicate, for which \(A=-BC\). Indeed, for blocks I and II, the estimate of the A main effect is \((a+abc)-(b+c)\) and for blocks III and IV it is \((ab+ac)-(bc+(1))\). Similarly, the estimates for B:C are \((a+abc)-(b+c)\) and \((bc+(1))-(ab+ac)\), respectively. Note that these estimates are all free of block effects. Then, the estimates of the two effects are also free of block effects and are proportional to \(\left[(a+abc)-(b+c)\right]\, +\, \left[(ab+ac)-(bc+(1))\right] = (a+ab+ac+abc)-((1)+b+c+bc)\) for A, respectively \(\left[(a+abc)-(b+c)\right]\, -\, \left[(ab+ac)-(bc+(1))\right]=((1)+a+bc+abc)-(b+c+ab+ac)\) for B:C. These are the same estimates as for two-fold replicate of the full factorial design. Somewhat simplified: the first two blocks allow estimation of \(A+BC\), the second pair allows estimation of \(A-BC\), the sum of the two is \(2\cdot A\), while the difference is \(2\cdot BC\).
The same argument does not hold for the A:B:C interaction, of course. Here, we have to contrast observations in \(ABC=+1\) blocks with observations in \(ABC=-1\) blocks, and block effects do not cancel. If instead of four blocks, our design only uses two blocks—one for each generator—then main effects and two-way interactions can still be estimated, but the three-way interaction is completely confounded with the block effect.
Using a classical ANOVA for the analysis, we indeed find two error strata for the inter- and intra-block errors, and the corresponding \(F\)-test for A:B:C in the inter-block stratum with two denominator degrees of freedom: we have four blocks, and loose one degree of freedom for the grand mean, and one degree of freedom for the A:B:C parameters. All other tests are in the intra-block stratum and based on six degrees of freedom: a total of \(4\times 4=16\) observations, with seven degrees of freedom spent on the model parameters except the three-way interaction, and three degrees of freedom spent on the block effects.
In summary, we can exploit the factorial treatment structure to our advantage when blocking, with only slightly more complex logistics to organize different treatment combinations for different blocks. Using a generator and its fold-over to alias a high-order interaction with the block effect, we achieve precise estimation of all effects not aliased with the block effect, and we can estimate the confounded effect with sufficient number of blocks based on the inter-block information.
9.8.3 Excursion: split-unit designs
While using the highest-order interaction to define the confounding with blocks is the natural choice, we could also use any other generator. In particular, we might use \(A=+1\) and \(A=-1\) as our two generators, thereby allocating half the blocks to the low level of A, and the other half to its high level. In other words, we randomize A on the block factor, and the remaining treatment factors are randomized within each block. This is precisely the split-unit design with the blocking factor as the whole-unit factor, and A randomized on it. With four blocks, we need one degree of freedom to estimate the block effect, and the remaining three degrees of freedom are split into estimating the A main effect (1 .d.f) and the between-block residual variance (2 d.f.). All other treatment effects profit from the removal of the block effect and are tested with 6 degrees of freedom for the within-block residual variance.
The use of generators offers more flexibility than a split-unit design, because it allows us to confound any effect with the blocking factor, not just a main effect. Whether this is an advantage depends on the experiment: if application of the treatment factors to experimental units is equally simple for all factors, then it is usually more helpful to confound a higher-order interaction with the blocking factor. This design then allows estimation of all main effects and their contrasts with equal precision, and lower-order interaction effects can also be estimated precisely. A split-unit design, however, offers advantages for the logistics of the experiment if levels of a treatment factor are more difficult to change than levels of the other factors. By confounding the hard-to-change factor with the blocking factor, the experiment becomes easier to implement. Split-unit designs are also conceptually simpler than confounding of interaction effects with blocks, but that should not be the sole motivation for using them.
9.8.4 Half-fraction with multiple generators
We are often interested in all effects of a factorial treatment design, especially if this design has only few factors. Using a single generator and a fold-over, however, provides much lower precision for the corresponding effect, which might be undesirable. An alternative strategy is then to use different generators and fold-overs for different pairs of blocks. In this partial confounding of effects with blocks, we confound a different effect in each pair of blocks, but can estimate the same effect with high precision from observations in the remaining blocks.
For example, we consider again the half-replicate of a \(2^3\)-factorial, with four units per block. If we have resources for 32 units in eight blocks, we can form four pairs of blocks with four units each. Then, we might use the previous generator \(G_1: ABC=\pm 1\) for our first pair of blocks, the generator \(G_2: AB=\pm 1\) for the second pair, \(G_3: AC=\pm 1\) for the third pair, and \(G_4: BC=\pm 1\) for the fourth pair of blocks. Each pair of blocks is then a fold-over pair for a specific generator with treatment combinations assigned as follows:
Block | Generator | 1 | 2 | 3 | 4 |
---|---|---|---|---|---|
I | ABC=+1 | a | b | c | abc |
II | ABC=-1 |
|
ab | ac | bc |
III | AB=+1 |
|
c | ab | abc |
IV | AB=-1 | a | b | ac | bc |
V | AC=+1 |
|
b | ac | abc |
VI | AC=-1 | a | b | ab | bc |
VII | BC=-1 |
|
a | bc | abc |
VIII | BC=+1 | b | c | ab | ac |
Looking at the resulting ANOVA table, we clearly see how information about effects is present both between and within blocks. Effects occurring in a generator are now present both in the inter-block error stratum and the residual (intra-block) error stratum:
##
## Error: block
## Df Sum Sq Mean Sq F value Pr(>F)
## A:B 1 0.3326 0.3326 0.906 0.411
## A:C 1 0.0031 0.0031 0.008 0.933
## B:C 1 0.7798 0.7798 2.125 0.241
## A:B:C 1 0.0021 0.0021 0.006 0.944
## Residuals 3 1.1011 0.3670
##
## Error: Within
## Df Sum Sq Mean Sq F value Pr(>F)
## A 1 0.977 0.9769 1.712 0.2082
## B 1 2.196 2.1956 3.847 0.0664 .
## C 1 1.587 1.5874 2.782 0.1137
## A:B 1 0.116 0.1155 0.202 0.6584
## A:C 1 0.100 0.1004 0.176 0.6801
## B:C 1 0.095 0.0946 0.166 0.6889
## A:B:C 1 0.220 0.2203 0.386 0.5426
## Residuals 17 9.701 0.5707
## ---
## Signif. codes: 0 '***' 0.001 '**' 0.01 '*' 0.05 '.' 0.1 ' ' 1
In this design each two-way interaction can be estimated using with-block information of three pairs of blocks, and the same is true for the three-way interaction. Additional estimates can be defined based on the inter-block information, similar to a BIBD. The inter- and intra-block estimates can be combined, but this is rarely done in practice for a classic ANOVA, where the more precise within-block estimates are often used exclusively. In contrast, linear mixed model offer a direct way of basing all estimates on all available data; a corresponding model for this example is specified as y~A*B*C+(1|block)
.
9.8.5 Multiple aliasing
We can further reduce the required block size by considering higher fractions of a factorial. As we saw in Section 9.5, these require several simultaneous generators, and additional aliasing occurs due to the generalized interaction between the generators.
For example, the half-fraction of a \(2^5\)-factorial still requires a block size of 16, which might not be practical. We further reduce the block size using the two pairs of generators \[ ABC=\pm 1\,,\quad ADE=\pm 1\,, \] with generalized interaction \(ABC\cdot ADE=BCDE\), leading to a \(2^{5-2}\) treatment design (Finney 1955, p101). Each of the four combinations of these two pairs selects eight of the 32 possible treatment combinations and a single replicate of this design requires four blocks:
Block | Generator | 1 | 2 | 3 | 4 | 5 | 6 | 7 | 8 |
---|---|---|---|---|---|---|---|---|---|
I | ABC=-1, ADE=-1 |
|
bc | de | bcde | abd | acd | abe | ace |
II | ABC=+1, ADE=-1 | b | c | bde | cde | ad | abcd | ae | abce |
III | ABC=+1, ADE=-1 | d | bcd | e | bce | ab | ac | abde | acde |
IV | ABC=+1, ADE=+1 | bd | cd | be | ce | a | abc | ade | abcde |
In this design, the two three-way interactions A:B:C and A:D:E, and the four-way interaction B:C:D:E used in the generators are partially confounded with block effects. All other effects, and in particular all main effects and all two-way interactions, are free of block effects and estimated precisely. By carefully selecting the generators, we are often able to confound effects that are known to be of lesser interest to the researcher.
Similar partially confounded designs exist for higher-order factorials. A prominent example is a \(2^{7-4}\) design that allows block sizes of eight instead of 128 and requires eight blocks for on replicate. The \(2^{7-4}\) fractional factorial has resolution III and thus confounds main effects with two-way interactions. By choosing the three generators intelligently, however, the partial confounding with blocks leaves main effects and two-way interaction confounded only with three-way and higher-order interactions.
9.8.6 Example: proteomics experiment
As a concrete example of blocking a factorial design, we discuss a simplified variant of a proteomics study in mice. The main target of this study is the response to inflammation, and a drug is available to trigger this response. One pathway involved in the response is known and many of the proteins involved as well as the receptor upstream of the pathway have been identified. However, related experiments suggested that the drug also activates alternative pathways involving other receptors, and one goal of the experiment is to identify proteins involved these pathways.
The experiment has three factors in a \(2^3\)-factorial treatment design: administration of the drug or a placebo, a short or long waiting time between drug administration and measurements, and the use of the wild-type or a mutant receptor for the known pathway, where the mutant inhibits binding of the drug and hence deactivates the pathway.
Expected results
We can broadly distinguish three classes of proteins that we expect to find in this experiment.
The first class are proteins directly involved in the known pathway. For these, we expect low levels of abundance for a placebo treatment, because the placebo does not activate the pathway. For the drug treatment, we expect to see high abundance in the wild-type, as the pathway is then activated, but low abundance in the mutant, since the drug cannot bind to the receptor and thus pathway activation is impeded. In other words, we expect a large genotype-by-drug interaction.
The second class are proteins in the alternative pathway(s) activated by the drug but exhibiting a different receptor. Here, we would expect to see high abundance in both wild-type and mutant for the drug treatment and low abundance in both genotypes for a placebo treatment, since the mutation does not affect receptors in these pathways. This translates into a large drug main effect, but no genotype main effect and no genotype-by-drug interaction.
The third class are proteins unrelated to any mechanisms activated by the drug. Here, we expect to see the same abundance levels in both genotypes for both drug treatments, and no treatment factor should show a large and significant effect.
We are somewhat unsure what to expect for the duration. It seems plausible that a protein in an activated pathway will show lower abundance after longer time, since the pathway should trigger a response to the inflammation and lower the inflammation. This would mean that a three-way interaction exists at least for proteins involved in the known or alternative pathways. A different scenario results if one pathway takes longer to activate than another pathway, which would present as a two- or three-way interaction of drug and/or genotype with the duration.
References
Davies, O. L. 1954. “The Design and Analysis of Industrial Experiments.” In. Oliver & Boyd, London.
Finney, David J. 1955. Experimental Design and its Statistical Basis. The University of Chicago Press.
Lenth, Russell V. 1989. “Quick and easy analysis of unreplicated factorials.” Technometrics 31 (4): 469–73. https://doi.org/10.1080/00401706.1989.10488595.
Plackett, R L, and J P Burman. 1946. “The design of optimum multifactorial experiments.” Biometrika 33 (4): 305–25. https://doi.org/10.1093/biomet/33.4.305.
It later transpired that the low level of N2 was zero in the first, but a low, non-zero concentration in the second replicate.↩
The NIST provides helpful designs on their website http://www.itl.nist.gov/div898/handbook/pri/section3/pri3347.htm.↩
http://www.itl.nist.gov/div898/handbook/pri/section3/pri335.htm↩